Demystifying Decision-making AI and Explainable AI (XAI)
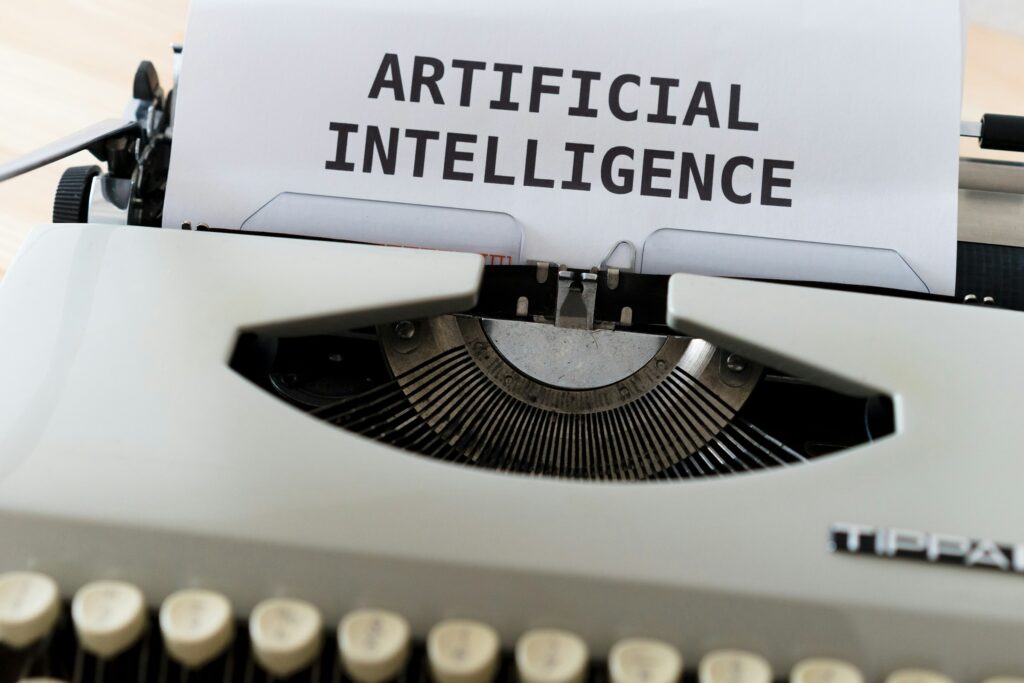
“Transparency and trust are the cornerstones of ethical AI. In the world of artificial intelligence, Decision-making AI shapes outcomes, while Explainable AI illuminates the path, ensuring accountability and understanding.” – Shree Shambav.
Understanding the Future of AI Technology
“Transparency and trust are the cornerstones of ethical AI. In the world of artificial intelligence, Decision-making AI shapes outcomes, while Explainable AI illuminates the path, ensuring accountability and understanding.” – Shree Shambav.
Artificial intelligence (AI) has transformed industries by enhancing processes and decision-making. Among the key concepts in AI are Decision-making AI and Explainable AI (XAI). Let’s delve into their meanings, significance, and impacts on the future of AI technology.
Decision-making AI:
Objective: Decision-making AI focuses on making accurate predictions or decisions based on input data.
Functionality: These AI systems process data, learn patterns, and make decisions or predictions without necessarily providing detailed explanations for their decisions.
Example: Consider a recommendation system like Netflix’s, which suggests movies or shows based on users’ past viewing behaviour.
Decision-making AI encompasses systems and algorithms designed to autonomously make decisions or recommendations based on data inputs. These systems analyse vast data sets, identify patterns, and make predictions or choices without human intervention. They find applications across domains like finance, healthcare, marketing, and autonomous vehicles.
While Decision-making AI excels in processing complex data quickly and efficiently, it raises concerns about transparency, accountability, and potential biases. As these systems often operate as black boxes, understanding their decision-making process poses challenges, leading to issues of trust and ethical implications. This is where Explainable AI comes into play.
Explainable AI (XAI):
Objective: XAI aims to provide transparency and interpretability in the decision-making process of AI systems.
Functionality: XAI techniques explain AI decisions or predictions in a human-understandable manner, providing insights into the factors influencing a decision.
Example: An AI system that predicts loan approval not only provides a decision, but also explains the relevant factors such as income and credit history.
Explainable AI (XAI) ensures AI systems can justify their decisions in a human-understandable manner, fostering transparency, interpretability, and trust. This is crucial in high-stakes domains like healthcare and criminal justice. XAI techniques include visualizing model internals, generating explanations for predictions, and quantifying feature impacts.
Conclusion:
Decision-making AI and Explainable AI are pivotal in shaping AI technology’s future. While Decision-making AI enables data-driven decision-making, Explainable AI ensures transparency and accountability in AI systems. Combining their capabilities creates powerful, trustworthy, and ethically sound AI systems, fostering responsible AI deployment across various domains.
In summary, while Decision-making AI produces accurate outcomes, Explainable AI ensures transparency and provides understandable explanations for those outcomes. XAI helps stakeholders understand and trust AI systems, promoting accountability and ethical use of AI technologies.